The beginning of the path to H100
The 5 miracles of Tesla P100 that set the foundations of the evolution of NVIDIA gpu cards focused on AI
AIHARDWARE COMPUTERSTECHNOLOGY
Eduardo Domínguez Menéndez
7/19/20243 min read
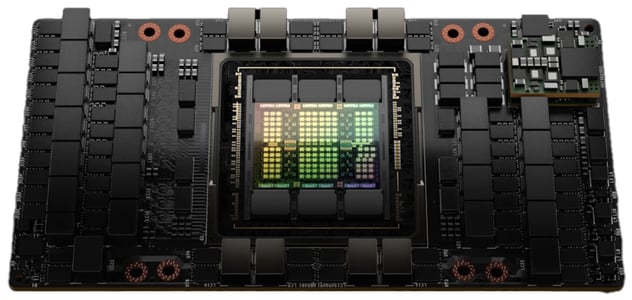
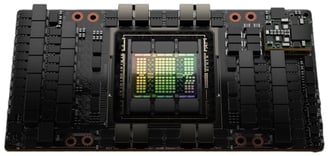
This is the first article of a serie where I explore the transformative shift of NVIDIA's core business from visual graphics to the forefront of AI, highlighted by the highly sought-after H100 as a symbol of the AI revolution, thanks to NVIDIA's groundbreaking hardware and CUDA software.
The turning point can arguably be pinpointed to the GPU Technology Conference (GTC) 2016 event, where Jensen Huang, the founder and CEO of NVIDIA, made a pivotal declaration: "we decided to be all in on AI".
This commitment was made just moments before unveiling the Tesla P100 GPU, a strategic move that laid the foundation for future architectures and successfully transitioned NVIDIA's core business from not just gaming and professional graphics but towards AI and High-Performance Computing (HPC). This transition fueled the AI revolution that unfolded in the years that followed.
If you or me had placed their bets on this vision back then with just $100 would see that investment grow to approximately $7500 by beginning of 2024. The "all in" stance by Jensen Huang, moments before introducing the Tesla P100, was a bold gamble that paid off, establishing the pillars for the development of upcoming architectures and making the shift towards AI and HPC a resounding success.
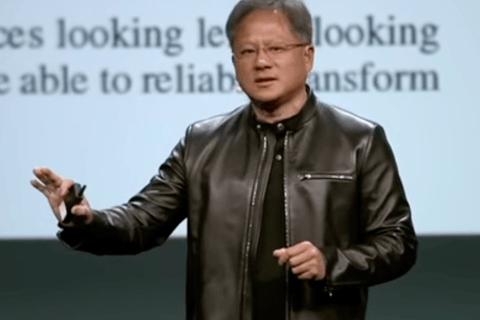
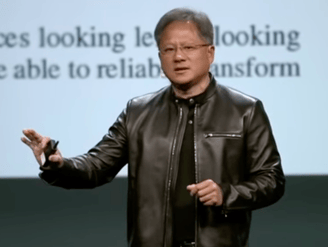
The Tesla P100 was heralded as an engineering and design triumph, challenging the company's internal development rules by tackling five technological challenges instead of the usual three. Huang described these challenges as the "five miracles," highlighting the groundbreaking nature of the P100.
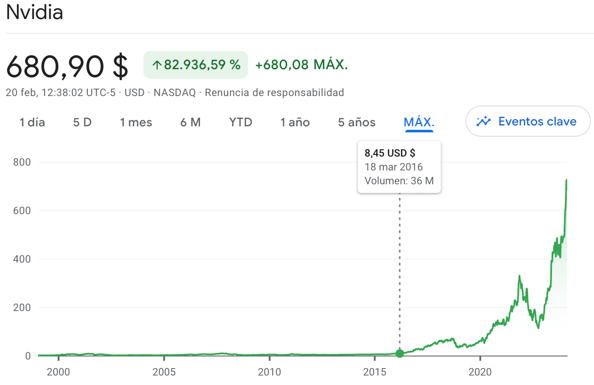
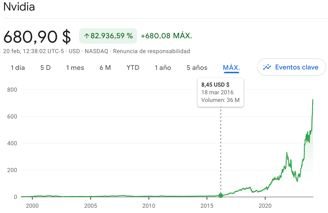
NVLink stands out as the Tesla P100's most disruptive innovation. Introduced at GTC 2014 as a teaser of the Pascal Architecture and its successors. Let's see state of the art at 2016: A PCI Express 3.0 had a throughput of 15.754 GB/s which limited bandwidth significantly compared to CPU and GPU memory speeds that could be estimated around 72 GB/s and 288 GB/s respectively. NVLink offered 80 GB/s, hence it effectively removed the bottleneck presented by PCI Express 3.0.
But this speed transfer enabled features like Unified Memory in CUDA 6 and facilitating direct GPU-GPU transfers and access, significantly improving data transfer rates and the efficiency of deep learning networks. In such tasks, NVLink achieved a 5 to 12 times increase in speed,
CoWoS with HBM2 technology represented another leap forward, addressing the challenges of signal speed, power efficiency, and performance in AI and HPC applications by integrating multiple chips on a single package for more efficient communication. HBM2, or High Bandwidth Memory second generation, further enhanced bandwidth and reduced the physical footprint on motherboards, crucial for compute-intensive applications. It is smaller so allows scale-out.
The introduction of the Pascal architecture marked a significant evolution from the previous Maxwell architecture, focusing on efficiency to meet the increasing demands of AI and HPC applications. With features like mixed-precision computing, NVIDIA significantly improved performance in deep neural network convolutions, paving the way for future advancements in AI.
The shift to FinFET 16 nm technology was another critical step, enabling NVIDIA to increase transistor density, reduce power consumption, and improve performance. This technology set the stage for future developments in NVIDIA's GPU families, including Volta, Ampere, and beyond. Other feature that allows scale-out.
Furthermore, the evolution of AI algorithms has been instrumental in leveraging the potential of NVIDIA's GPUs, with new algorithms being developed to maximize the architectural advancements. Yann LeCun, consider one of the "Godfathers" of the AI, noted NVIDIA's GPUs as pivotal in accelerating progress in AI, emphasizing the need for faster GPUs, quicker GPU-to-GPU communication, and hardware capable of reduced-precision arithmetic, all delivered by the Pascal architecture.
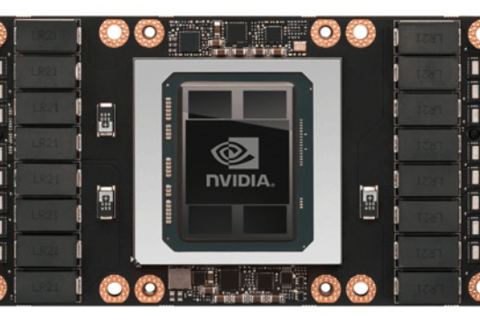
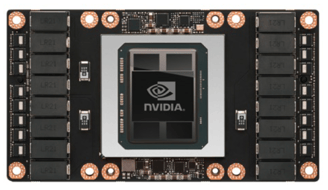
Jensen Huang pointed at GTC 2016 the following quote from Yann LeCun: "NVIDIA GPU is accelerating progress in Al. As neural nets become larger and larger, we not only need faster GPUs with larger and faster memory, but also much faster GPU-to-GPU communication, as well as hardware that can take advantage of reduced-precision arithmetic. This is precisely what Pascal delivers."
You can watch the video version of this article here.
The next articles of this serie will dive in the five miracles that enable the Tesla P100
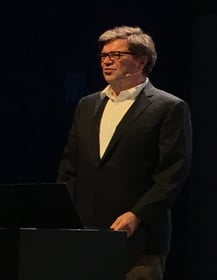
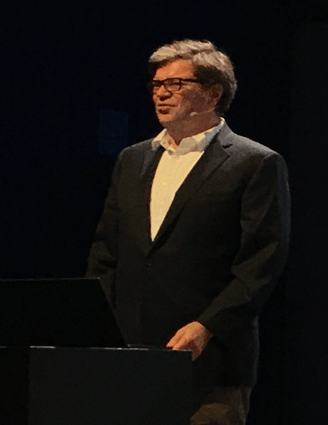